The Next Era of AI: OpenAI and Rivals Forge New Paths as Scaling Reaches Its Limits
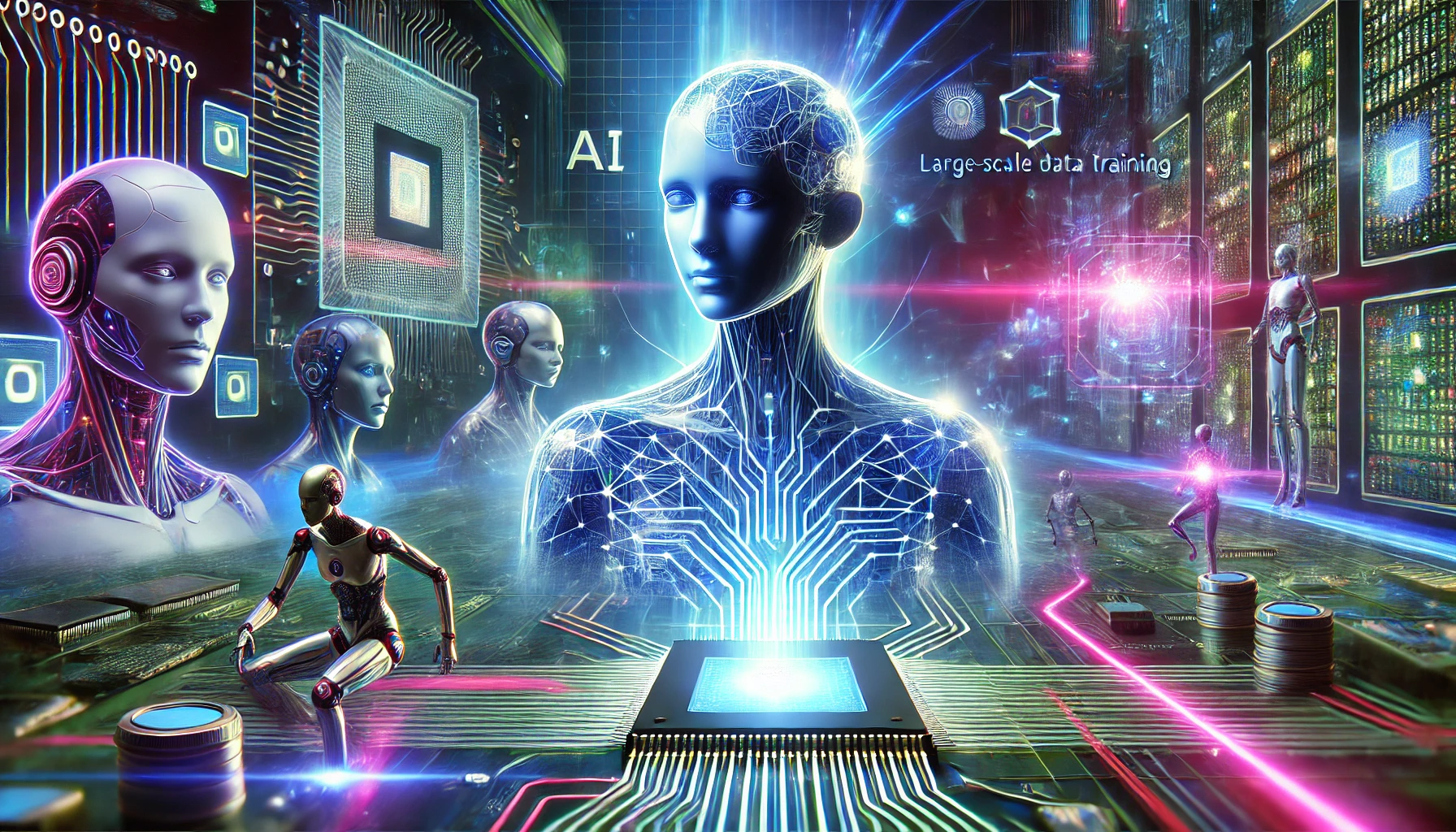
The quest for smarter artificial intelligence (AI) is shifting. No longer is “bigger is always better” the prevailing mantra in the race to develop advanced AI systems. Instead, researchers and AI companies like OpenAI are reevaluating their approach to building large language models (LLMs). The reason? The traditional path of expanding models by endlessly scaling data and computational power has hit serious roadblocks.
The Challenge of Scaling Large Language Models
In recent years, scaling up AI has yielded impressive results. Models like OpenAI’s GPT-4 achieved groundbreaking success by using vast amounts of data and powerful computing resources. However, this success is not without cost. The so-called “training runs” for these models are costly, requiring tens of millions of dollars and immense amounts of energy. These runs involve hundreds of AI chips operating simultaneously, making them prone to hardware-induced failures and lengthy, unpredictable training times. Moreover, AI models have nearly exhausted easily accessible data sources, raising questions about the feasibility of further scaling.
According to Ilya Sutskever, co-founder of Safe Superintelligence (SSI) and a former OpenAI leader, the era of simply building ever-larger models has passed. “The 2010s were the age of scaling; now we’re back in the age of wonder and discovery once again,” Sutskever told Reuters. While he and other experts seek new ways to push AI forward, the challenges of high computational costs and power constraints have made clear that the future requires fresh approaches.
The Emergence of “Test-Time Compute”
To address these limitations, researchers are turning their attention to “test-time compute,” a method that focuses on enhancing AI models during the inference phase. In this context, inference refers to the model’s use and performance after training, such as answering questions, generating text, or making predictions. The idea is simple but powerful: instead of training a model endlessly on vast datasets, use smarter computational strategies to improve its reasoning capabilities in real-time.
By dedicating more processing power to difficult tasks like solving complex math problems or making human-like decisions, models can mimic thoughtful human deliberation. This technique enables AI systems to generate multiple potential solutions and select the best one, much like weighing options before making a decision.
OpenAI’s O1 Model and the Race for Smarter AI
OpenAI’s recently released O1 model, formerly codenamed Q* and Strawberry, exemplifies this shift. Unlike its predecessors, the O1 model “thinks” through problems in multiple steps, much like a human would. This new approach involves supplementing base models like GPT-4 with additional training guided by industry experts and researchers.
During a TED AI conference, Noam Brown, an OpenAI researcher, explained the potential of test-time compute: “It turned out that having a bot think for just 20 seconds in a hand of poker got the same boosting performance as scaling up the model by 100,000x and training it for 100,000 times longer.” Such advancements demonstrate that smarter, rather than merely larger, models may represent the future of AI development.
Implications for AI Hardware and Competition
The shift from massive pre-training models to more efficient inference-based models could reshape the AI hardware market. Until now, Nvidia has dominated AI chip production, capitalizing on the demand for training chips used in large-scale models. However, as AI companies pivot toward distributed inference servers, Nvidia could face increased competition in this emerging market.
Sequoia Capital partner Sonya Huang remarked that “this shift will move us from a world of massive pre-training clusters toward inference clouds.” As more AI firms adopt test-time compute and distributed inference strategies, the demand for hardware optimized for inference will likely increase, providing opportunities for new market players.
A Competitive AI Landscape Awaits
The drive for smarter AI isn’t limited to OpenAI. Other leading AI labs, including Anthropic, Google DeepMind, and xAI, are reportedly developing their versions of these methods. The competition remains fierce, but the focus has evolved. The companies that master test-time compute, more efficient inference, and innovative scaling techniques may define the next phase of AI’s evolution.
OpenAI’s Kevin Weil summed up the challenge well, stating, “By the time people do catch up, we’re going to try and be three more steps ahead.” As AI researchers explore new methods to boost intelligence without ballooning computational costs, one thing is certain: the era of AI optimization has just begun.