AI Winter: A Cycle of Hype, Disappointment, and Recovery ❄️🤖
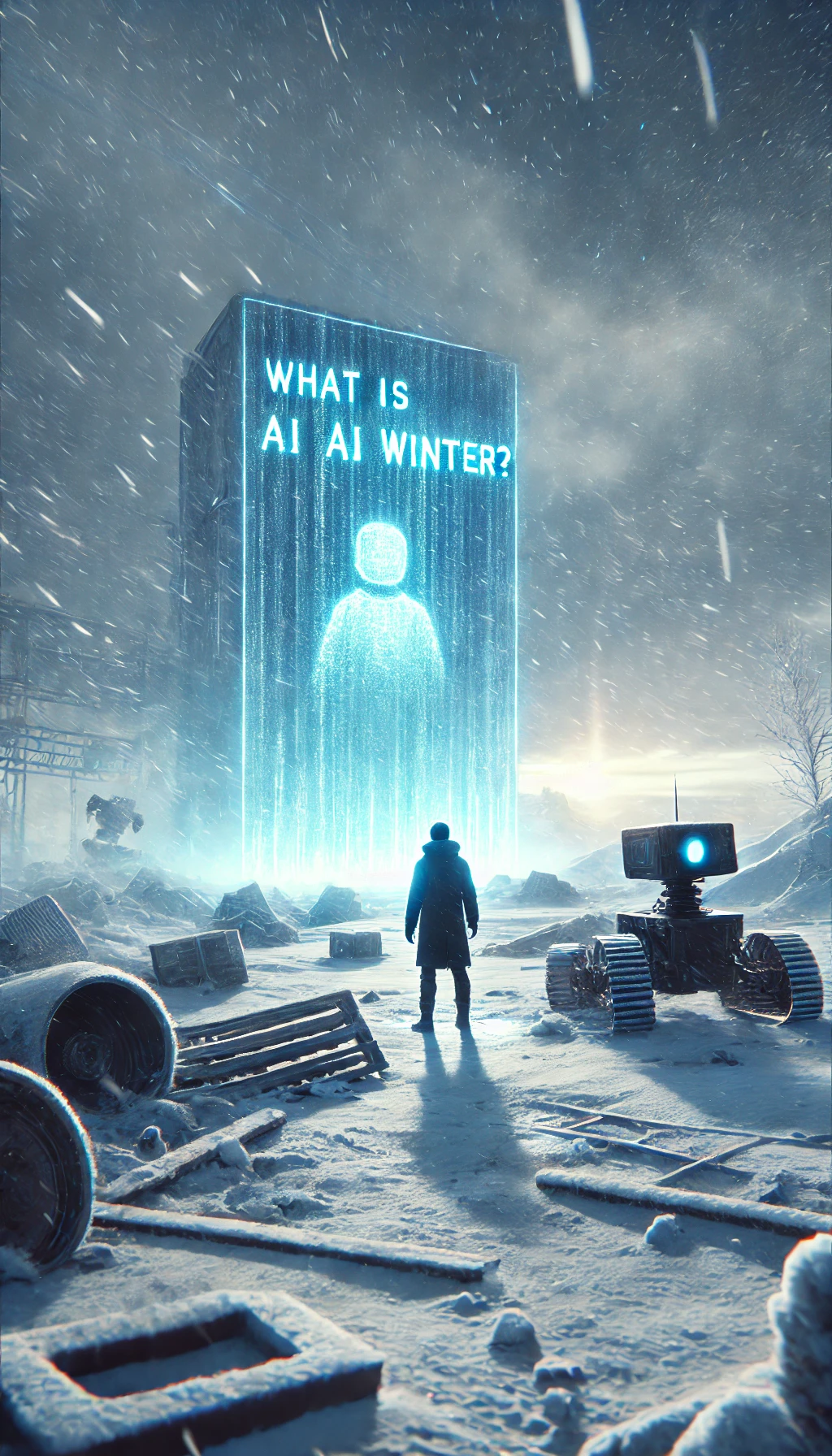
By Muhammad Zulhusni | September 9, 2024
Categories: Applications, Artificial Intelligence, Cloud, Industries, Machine Learning, Research
Artificial Intelligence (AI) has long captivated the imagination of researchers, businesses, and the general public alike. But history tells us that the journey of AI is not a straightforward ascent toward technological dominance. Instead, it is marked by periods of hype, followed by crushing disappointment — commonly known as AI winters.
What Is AI Winter? 🧊
An AI winter occurs when the excitement and overblown promises of AI technology fail to deliver tangible results. This often leads to a loss of investor confidence, research funding cuts, and a general cooling off of interest in AI. Essentially, AI winters represent periods of stagnation for the field.
A Familiar Pattern of Hype and Letdown
Generative AI, like OpenAI’s GPT-4o and Google’s AI-powered overviews, has recently reignited this cycle. While there was enormous hype surrounding the potential of these technologies, many have fallen short of expectations, echoing a pattern seen before.
The first AI winter hit in the 1970s when overly ambitious projects aimed at solving machine translation and speech recognition ran up against technical limitations. Expectations were sky-high, but the technology wasn’t there yet. As a result, funding froze, leaving the AI field in a cold lull.
The Second AI Winter of the 1980s: A Deeper Freeze 🌨️
The 1980s saw renewed excitement with expert systems — AI that could mimic human decision-making. However, they, too, crumbled under real-world challenges, such as handling unexpected inputs. High-profile projects like Japan’s Fifth Generation Computer Project and the decline of LISP machines also contributed to the onset of the second AI winter.
As AI failed to meet expectations, the stigma surrounding the field grew. Many researchers distanced themselves from AI, choosing instead to focus on informatics or machine learning to avoid the negative connotations.
Surviving the Cold: AI’s Resilience 🛠️
Despite these harsh winters, AI research didn’t disappear. The 1990s were challenging for AI, with few practical implementations emerging. Even IBM’s famed Watson, once touted as a game-changer in medical diagnosis, faced significant real-world challenges. Watson struggled with interpreting doctors’ notes and adapting to local medical practices, exposing AI’s limitations in high-stakes environments.
The 2000s saw AI funding re-emerge, thanks to advances in machine learning and the availability of big data. However, investors remained cautious, often focusing on buzzwords like blockchain, autonomous vehicles, and voice-command devices, many of which eventually failed to meet inflated expectations.
What Can We Learn from Past AI Winters? 🌱
Each AI winter serves as a sobering reminder of the danger of overhyping technology. Unrealistic expectations not only hurt AI development but also cause deep financial repercussions, forcing researchers to pivot to smaller, short-term projects.
Here’s what history teaches us:
- Be realistic about AI’s capabilities: Don’t expect a revolution overnight. AI advances are slow and incremental.
- Focus on foundational research: Groundbreaking AI technologies take time to develop. Rushing leads to disappointment.
- Transparent communication with investors and the public: Set achievable goals and explain limitations upfront.
These lessons can help us mitigate the impact of future AI winters, ensuring we don’t repeat past mistakes.
Are We Headed Toward Another AI Winter? 🧐
After a blockbuster 2023 for AI, it seems like the momentum has slowed. Generative AI breakthroughs are becoming less frequent, and investors are growing more skeptical. Tools like ChatGPT, while impressive, have struggled to live up to their hype. The presence of AI hallucinations, where AI outputs false or nonsensical information, coupled with ethical concerns about data usage, are significant hurdles.
That said, it’s not all doom and gloom. Open-source models are emerging as strong contenders against closed alternatives, and companies are experimenting with AI in niche markets. Perplexity, for instance, has found a foothold in the search space, signaling that AI might avoid a complete freeze this time around.
The Future of AI and Business Impact 🚀
Where does that leave businesses? AI is here to stay, but its path forward is uncertain. Generative AI models need to overcome serious limitations before they can offer the productivity boosts promised. Issues surrounding data ethics, safety, and accuracy must also be addressed to maintain public and investor trust.
For businesses looking to leverage AI, the key will be authenticity and trust. AI tools must be used responsibly, with companies focusing on sustainable, long-term benefits rather than short-term hype. As search marketers and AI professionals, understanding AI’s current limits is essential. Experiment cautiously, aim for productivity gains, and avoid becoming overly reliant on a technology that’s still evolving.
Want to dive deeper into AI and emerging tech? Check out the AI & Big Data Expo in Amsterdam, California, and London, where industry leaders explore the future of AI and its business impact. 💡
Tags: Artificial Intelligence, Machine Learning, Research